近日,半岛·体育(BOB)中国官方网站人工智能与信息安全法律研究中心刘东亮教授指导的博士研究生张楚悦、孟宇辰在SSCI一区期刊《计算机法律与安全评论》(Computer Law & Security Review , Volume 55, November 2024)上发表论文《贝叶斯深度学习:与法律推理相协调的增强型人工智能框架》(Bayesian Deep Learning: An Enhanced AI Framework for Legal Reasoning Alignment)。
与当前学界的关注焦点集中于深度学习不同,文章认为,贝叶斯推理与法律人的思维过程高度契合。贝叶斯推理可以进行溯因推理,擅长因果推理,并承认推理结论的 “可废止性”,符合法律人从先验到后验的认知发展规律。基于贝叶斯方法的人工智能模型应当成为法律人工智能系统的主要技术支撑。贝叶斯神经网络在不确定性建模、避免过拟合、可解释性等方面具有优势。基于贝叶斯深度学习框架的法律人工智能系统可以结合深度学习和概率图模型的优势,促进感知任务和推理任务之间的信息交换和补充。本文以犯罪人预测系统和法律判决预测系统为例,探讨贝叶斯深度学习框架的构建和基本运行模式。贝叶斯深度学习可以增强推理能力,提高模型的可解释性,使推理过程更加透明和可视化。此外,贝叶斯深度学习框架非常适合人机协作任务,可以实现人机优势互补。
《计算机法律与安全评论》由英国信息技术法律与公共政策研究专家Steve Saxby教授于1985年创办,是以互联网法律、网络安全、数据保护、人工智能等科技与法律交叉研究为主要特色的著名国际学术期刊,是世界范围内科技法律期刊中为数不多入选SSCI目录的法学类期刊。
根据科睿唯安最新发布的2023年期刊引证报告(Journal Citation Reports, JCR),《计算机法律与安全评论》位列SSCI的Q1区序列,在共计430本SSCI法学刊物中位列第7位。
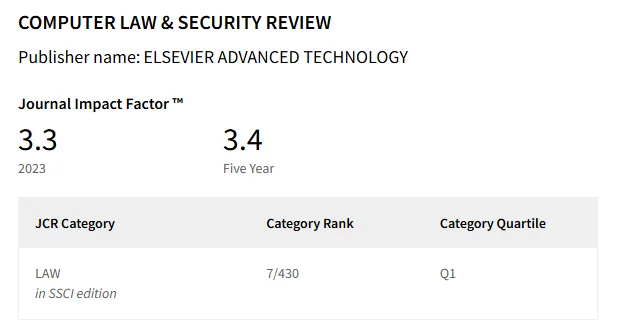
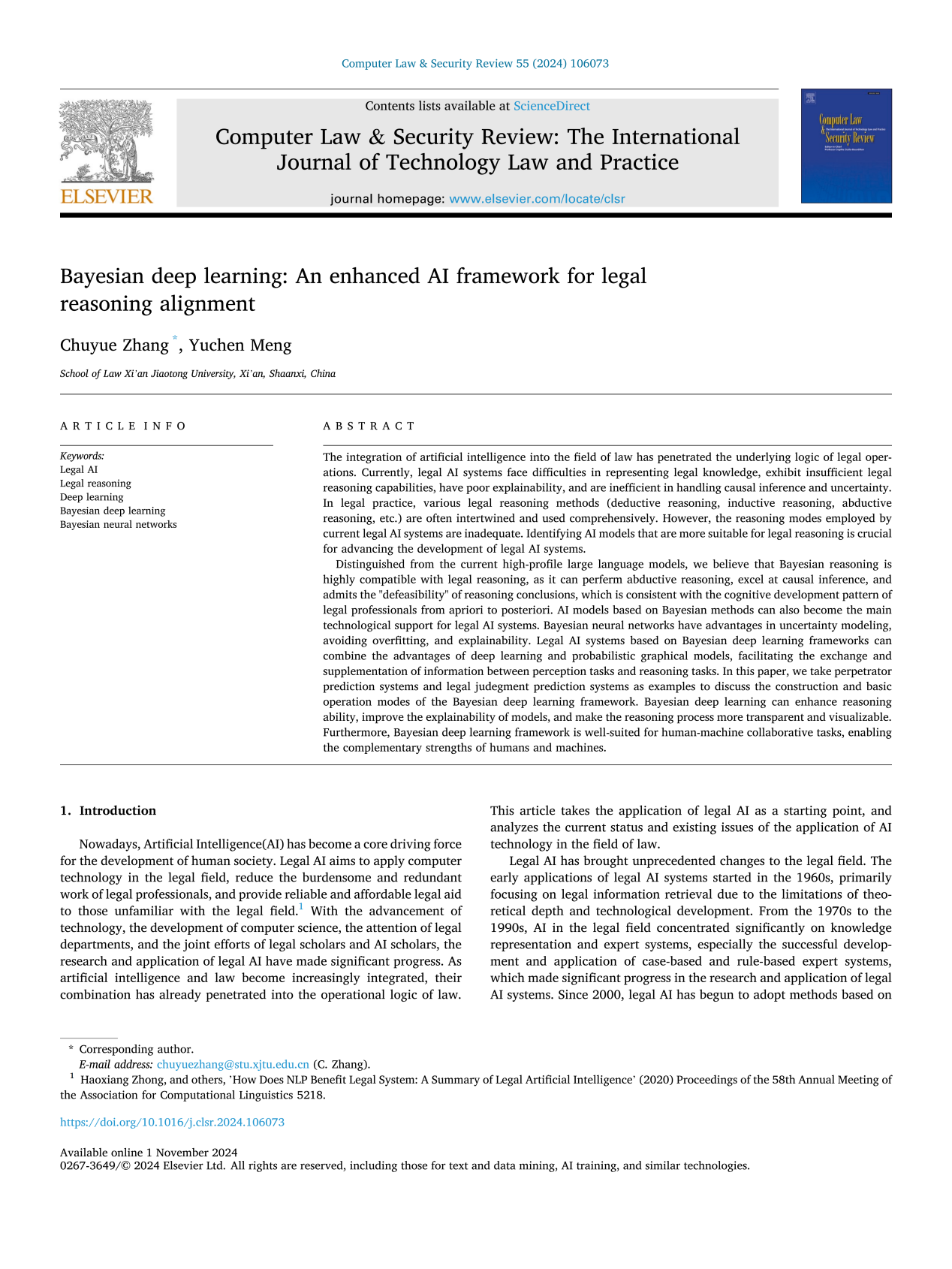
Abstract
The integration of artificial intelligence into the field of law has penetrated the underlying logic of legal operations. Currently, legal AI systems face difficulties in representing legal knowledge, exhibit insufficient legal reasoning capabilities, have poor explainability, and are inefficient in handling causal inference and uncertainty.
In legal practice, various legal reasoning methods (deductive reasoning, inductive reasoning, abductive reasoning, etc.) are often intertwined and used comprehensively. However, the reasoning modes employed by current legal AI systems are inadequate. Identifying AI models that are more suitable for legal reasoning is crucial for advancing the development of legal AI systems.
Distinguished from the current high-profile large language models, we believe that Bayesian reasoning is highly compatible with legal reasoning, as it can perferm abductive reasoning, excel at causal inference, and admits the "defeasibility" of reasoning conclusions, which is consistent with the cognitive development pattern of legal professionals from apriori to posteriori. AI models based on Bayesian methods can also become the main technological support for legal AI systems. Bayesian neural networks have advantages in uncertainty modeling, avoiding overfitting, and explainability. Legal AI systems based on Bayesian deep learning frameworks can combine the advantages of deep learning and probabilistic graphical models, facilitating the exchange and supplementation of information between perception tasks and reasoning tasks. In this paper, we take perpetrator prediction systems and legal judegment prediction systems as examples to discuss the construction and basic operation modes of the Bayesian deep learning framework. Bayesian deep learning can enhance reasoning ability, improve the explainability of models, and make the reasoning process more transparent and visualizable. Furthermore, Bayesian deep learning framework is well-suited for human-machine collaborative tasks, enabling the complementary strengths of humans and machines.
Keywords:Legal AI; Legal reasoning; Deep learning; Bayesian deep learning; Bayesian neural network
文章链接:https://www.sciencedirect.com/science/article/pii/S0267364924001390